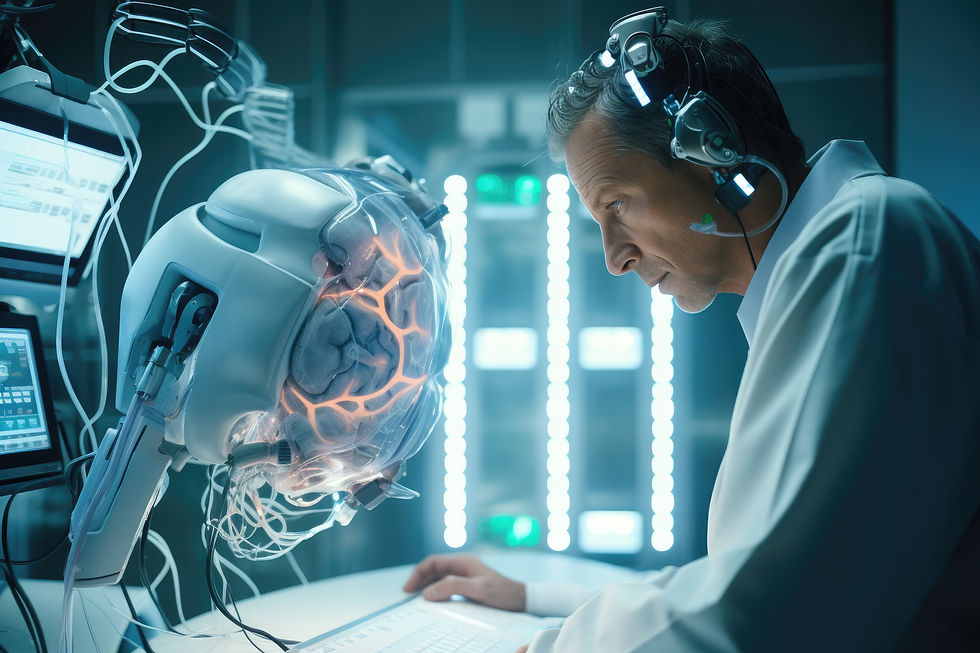
Manish Garg
Student, B.A. LL.B. (Hons.)
LEGAL HOUSE LAW JOURNAL - ISSN NO. - 3048-779X
ISSUE - I Volume - I
26 Jun 2024
School of Law, Sushant University
RESEARCH PAPER
1. ABSTRACT
By improving diagnostic precision, customizing care, and expediting the delivery of healthcare, artificial intelligence (AI) has the potential to completely transform the healthcare industry. This essay examines the historical development of artificial intelligence in the healthcare industry, looks at its present uses and problems, and looks ahead to potential advancements. It also explores the moral and legal issues related to artificial intelligence in healthcare.
By improving streamlining treatment plans, and completely changing the way patients are cared for, artificial intelligence (AI) is quickly changing the healthcare industry. This paper examines the development, uses, and potential consequences of artificial intelligence in healthcare.
Historically, artificial intelligence (AI) in healthcare started with neural networks and rule-based systems and progressed to complex machine learning algorithms and natural language processing. AI is currently used by doctors to support robotic surgery, customized medicine, and predictive analytics, all of which improve patient outcomes and operational efficiency.
In the future, AI promises to significantly transform healthcare through developments in genetically-based individualized treatment regimens, AI-driven diagnostics, and improved telemedicine capabilities. However, to fully realize AI's potential in healthcare, ethical issues, data privacy concerns, and regulatory obstacles must be overcome.
2. INTRODUCTION
In the field of healthcare, artificial intelligence (AI) has shown itself to be a revolutionary force, redefining the way doctors diagnose conditions, create treatment regimens, and provide patient care. Artificial intelligence (AI) technology have advanced over the past few decades from abstract ideas to real-world uses that have the potential to completely change the way healthcare is provided. This paper examines the development, use, and potential of artificial intelligence (AI) in healthcare, emphasizing how it can significantly enhance patient experiences, operational effectiveness, and medical results.The use of AI in healthcare represents a conceptual shift rather than just a technical development. It makes use of enormous data sets, complex algorithms, and computing power to supplement human judgment in clinical decision-making and healthcare administration.
Artificial intelligence (AI) has steadily improved diagnostic accuracy, individualized treatment plans, and operational procedures in healthcare settings. Examples of AI applications include early rule-based systems and neural networks in medical imaging, as well as modern machine learning algorithms and natural language processing. These days, AI systems quickly and accurately evaluate large, complex information to provide insights that help with early illness identification, patient outcome prediction via predictive analytics, and resource management for the healthcare industry. Furthermore, AI-driven advancements in customized medicine, robotic surgery, and virtual health assistants represent the continuous transition towards a healthcare ecosystem that is more patient-centered, effective, and sustainable.
In the future, artificial intelligence in healthcare could lead to even more breakthroughs. Future improvements are expected to include AI-driven diagnostics that can combine many data sources, more individualized treatment regimens based on patient histories and genetic profiles, and improved accessibility to healthcare via telemedicine and remote monitoring tools. But these prospects also bring with them a number of serious difficulties, such protecting patient privacy, reducing algorithmic bias, and negotiating legal frameworks that control the use of AI in healthcare. This study looks at various aspects of artificial intelligence's development in the healthcare industry, offering insights into how these technologies are influencing healthcare delivery now and in the future globally. .
3. HISTORICAL DEVELOPMENTS
Beginning in the middle of the 20th century, expert systems and early computing models that mimicked human decision-making were used in healthcare. Important turning points include the creation of systems like MYCIN in the 1970s, which set the foundation for more advanced AI technologies and were used to diagnose bacterial illnesses and suggest therapies.
3.1 Early AI in Medicine
Researchers started experimenting with artificial intelligence (AI) in medicine in the 1960s and 1970s, creating systems like DENDRAL and MYCIN. AI's promise in diagnostics was demonstrated by MYCIN, which was designed to detect bacterial illnesses and recommend therapies, and DENDRAL, which was developed at Stanford University for chemical analysis. Rule-based algorithms, which base choices on pre-established rules and conditions, were a major component of these early systems. These systems showed that artificial intelligence (AI) had the ability to support intricate decision-making processes, notwithstanding certain limits.
3.2 Machine Learning's Ascent
A branch of AI known as machine learning—which trains algorithms on massive datasets—rose to prominence in the ensuing decades. The power of AI to analyze and comprehend complicated medical data significantly advanced during this era with the development of
3.3 Big Data and AI
A data explosion occurred in the 21st century when electronic health records (EHRs) became widely used. This data explosion gave AI the fuel it needed to create increasingly sophisticated models. Multi-layered neural network techniques like deep learning started to show promise in jobs like natural language processing and picture recognition. Big data and AI integration made it possible for more thorough analysis and improved prediction skills, which resulted in more individualized and efficient healthcare solutions .
.
3.4 Contemporary AI Uses
AI has been essential to many healthcare applications in recent years. Modern AI is making a substantial contribution to healthcare, as demonstrated by systems like Google's DeepMind for retinal disease diagnosis and IBM Watson's Oncology platform, both of which have demonstrated their effectiveness in actual clinical situations. These sophisticated artificial intelligence systems.
4. LEGAL AND ETHICAL CONSIDERATIONS
With the widespread adoption of electronic health records (EHRs) in the twenty-first century, there was a data explosion. The surge of data provided AI with the fuel it required to build ever-more complex models. Deep learning and other multi-layered neural network techniques began to show promise in fields like picture recognition and natural language processing. Integration of big data with AI enabled more in-depth analysis and enhanced prediction abilities, leading to more customized and effective healthcare solutions.
4.1 Data Privacy and Security
Data privacy is one of the main issues with AI in healthcare. The substantial volumes of patient data needed to train AI systems present serious privacy concerns. It is crucial to guarantee data security and adherence to laws such as the Health Insurance Portability and Accountability Act (HIPAA) in the US. To stop unwanted access and data breaches, strong encryption techniques, safe data storage options, and stringent access controls must be put in place.
4.2 Algorithmic bias
Biases in training data are reflected in AI systems. An AI system will provide biased results if the data is prejudiced, which could result in unfair or erroneous consequences. Ensuring diverse training datasets and applying fairness-aware machine learning techniques are necessary steps in addressing algorithmic bias. AI systems must also be continuously updated and monitored.
4.3 Accountability and Transparency
It's difficult to assign blame for healthcare judgments made with AI. Determining who is responsible for a diagnostic error made by an AI system is difficult. This highlights the necessity for explainable AI and transparent AI algorithms so that humans can comprehend and examine the decision-making process. Creating accountability frameworks guarantees that regulatory agencies, healthcare providers, and AI developers all take responsibilities for AI results.
4.4 AI's Ethical Use
Beyond merely adhering to the law, ethical AI use in healthcare ensures that AI innovations improve patient care without taking the place of human judgment. Ensuring fair access to AI technologies is another ethical concern, as this will ensure that advantages are not restricted to affluent areas or populations. Encouraging the use of AI ethically entails creating rules for the appropriate application of AI systems, placing patient safety first, and upholding
5. CURRENT APPLICATIONS AND CHALLENGES
AI is being used in a number of healthcare applications, including drug development, genomics, medical imaging, and customized medicine. Radiologists may diagnose diseases earlier with the use of AI-powered diagnostic technologies, and patient outcomes and treatment are enhanced by predictive analytics. Nonetheless, there are still many obstacles to overcome, including issues with data quality, interaction with current healthcare systems, and the requirement for thorough validation and clinical studies.
5.1 Imaging in Medicine
In the field of medical imaging, AI has advanced significantly. Medical picture interpretation has shown algorithms, especially convolutional neural networks (CNNs), to be remarkably accurate. These algorithms can identify anomalies in CT, MRI, and X-ray scans with an accuracy that frequently equals that of human radiologists. AI in medical imaging improves diagnostic precision and expedites the analysis process, enabling prompt treatment decisions and improved patient outcomes .
5.2 Precision Medicine and Genomics
AI is revolutionizing genomics by accelerating the process of analyzing genetic data. Precision medicine, in which a patient's therapy is customized based on their genetic profile, relies on machine learning algorithms to find patterns and connections in genomic data. This individualized approach to healthcare lowers adverse responses and increases treatment efficacy
5.3 Drug Discovery
AI, which forecasts how substances will interact with human body targets, is streamlining the drug discovery process, which has historically been costly and time-consuming. Artificial intelligence (AI) systems find possible new medications more quickly than traditional approaches by analyzing enormous datasets of chemical compounds and biological data. In doing so, unmet medical needs are addressed and patient care is improved when novel medicines are developed and brought to market more quickly.
5.4 Individualized Healthcare
Artificial intelligence (AI) makes customized medicine possible by customizing treatment plans based on a patient's genetic information, lifestyle, and environmental circumstances. By taking into account each patient's unique variability, this method enhances treatment success and minimizes unwanted effects. By integrating many data sources, personalized medicine uses AI to provide a comprehensive picture of a patient's health and optimize treatment plans.
5.5 Remote Care and Telemedicine
AI's ability to provide remote monitoring and diagnosis is transforming telemedicine. Chatbots and virtual assistants driven by artificial intelligence (AI) streamline patient contacts, provide medical advice, and help with appointment scheduling. In order to check vital signs and identify early indicators of health problems, remote patient monitoring systems analyze data from wearable devices. This allows for prompt treatments and lowers the number of readmissions to hospitals.
5.6 Effectiveness of Administration
AI enhances administrative effectiveness in healthcare environments as well. By transcribing and summarizing clinical notes, natural language processing (NLP) algorithms simplify the documentation process and free up healthcare workers to concentrate on patient care. AI-powered scheduling solutions streamline the distribution of resources, cut down on wait times, and improve overall productivity.
5.7 Difficulties
Notwithstanding these developments, a number of obstacles prevent AI from being widely used in healthcare:
- 5.7.1 Data Quality and Quantity: Acquiring and curating high-quality, annotated data is a difficult task for AI models to be effective.
- 5.7.2 Integration with Healthcare Systems: AI systems need to work in unison with the current healthcare infrastructure, which frequently consists of intricate workflows and outdated systems.
- 5.7.3 Regulatory permission: Extensive validation and expensive clinical trials are required to obtain regulatory permission for AI systems in the healthcare industry.
- 5.7.4 Interdisciplinary Collaboration: It can be difficult to coordinate the cooperation that AI experts, healthcare professionals, and regulatory agencies need in order to use AI in healthcare effectively.
- 5.7.5 Trust and Acceptance: It's critical to foster patient and healthcare provider trust in AI. For AI to be widely accepted, open communication about its advantages and disadvantages is crucial, as is showcasing the technology's effectiveness through clinical studies.
6. AI'S FUTURE IN HEALTHCARE
With developments in deep learning, natural language processing, and robotics, artificial intelligence in healthcare appears to have a bright future. AI is anticipated to be a key component of precision medicine, allowing for highly customized treatment regimens based on a patient's genetic composition and way of life. AI may also revolutionize telemedicine by enabling remote diagnosis and treatment, especially in underprivileged areas.
6.1 Deep Learning Advancements
Healthcare is expected to develop significantly due to machine learning, namely in the area of deep learning. Deep learning algorithms are able to reveal insights that were previously unattainable by examining vast and intricate datasets.
Capable. Upcoming uses include real-time patient health monitoring, tailored therapy suggestions, and early disease identification 6.2 Analysis of Natural Language.
AI systems will be able to comprehend and analyze clinical notes, medical literature, and patient interactions more effectively thanks to advances in natural language processing (NLP). In the end, this will result in greater patient care and operational efficiency by streamlining administrative processes, enhancing patient-physician communication, and improving decision support systems.
6.3 AI and Robotics
AI combined with robotics will transform patient care, rehabilitation, and surgery. Robots driven by artificial intelligence (AI) can help in minimally invasive procedures by providing accuracy and speeding up recuperation. Robotic exoskeletons in rehabilitation can help patients move around and heal, while AI-powered robots can help elderly and crippled patients by becoming a friend and helper.
6.4 AI in the Management of Population Health
AI's ability to recognize patterns and forecast health outcomes for big populations can have a substantial impact on population health management. In the end, this improves general public health and lowers healthcare costs by enabling preventive treatments, focused public health campaigns, and optimal resource allocation.
6.5 AI in Psychology
Artificial Intelligence (AI) holds great promise in the field of mental health care since it can offer tailored treatment programs, track patient progress, and anticipate possible mental health emergencies. Artificial intelligence (AI)-powered apps can provide assistance through online counseling sessions, mood monitoring, and tailored advice, improving the effectiveness and accessibility of mental health services.
6.6 Utilizing Emerging Technologies in Integration
Healthcare will have new opportunities as a result of AI's integration with cutting-edge technologies like blockchain, augmented reality, and the Internet of Things. Blockchain technology can provide safe and open health data management, while IoT devices can feed AI systems with real-time health data for ongoing monitoring. AI and AR together can improve patient care, diagnosis, and medical education.
6.7 AI in Global Health
AI can solve problems with global health by offering scalable and affordable solutions. Predictive analytics can assist control disease outbreaks and optimize resource allocation, while AI-powered diagnostic tools and telemedicine platforms can enhance access to healthcare in low-resource environments.
7. ADVANCED AI RECOMMENDATIONS FOR HEALTHCARE
7.1 Improving Accessibility and Data Quality
It's critical to improve data accessibility and quality in order to realize AI's full potential in healthcare. This entails putting in place strong data governance frameworks, guaranteeing system compatibility, and standardizing data formats. Effective AI models are trained on high-quality data, and data accessibility guarantees that data can be used for development, research, and clinical applications.
7.2 Encouraging Multidisciplinary Coordination
Successful application of AI in healthcare requires cooperation between regulatory agencies, medical experts, and AI researchers. Multidisciplinary teams make sure AI systems are rigorously tested and designed to satisfy clinical needs. Encouraging communication and cooperation across these disparate groups can hasten the advancement and uptake of artificial intelligence technologies.
7.3 Handling Legal and Ethical Issues
Creating thorough policies and rules for the moral application of AI in healthcare is imperative in order to tackle concerns about algorithmic bias, data privacy, and responsibility. Establishing trust between patients and healthcare practitioners should be a top priority for transparent and explainable AI systems. Involving patients and other stakeholders in the formulation of these recommendations guarantees that a variety of viewpoints are taken into account.
7.4 Putting Money Into Training and Education
To properly deploy AI technologies, healthcare workers need to possess the necessary knowledge and abilities. Putting money into educational and training initiatives helps ensure that healthcare professionals can use AI to enhance patient care by bridging the knowledge gap between the field and clinical practice. The practical use of AI in clinical settings should be the core emphasis of training programs, along with the significance of upholding ethical norms.
7.5 Promoting Research and Innovation
To enhance healthcare, more money must be invested in AI research and innovation. Governments, educational institutions, and private businesses ought to work together to
finance research projects and encourage the creation of cutting-edge AI applications. AI-driven healthcare innovations may advance if an innovative culture is promoted and resources are made available for testing.
7.6 Guaranteeing Fair Access
It is important to make sure that everyone, especially those living in underprivileged and low-resource environments, may benefit from AI in healthcare. Policies that address inequities in healthcare delivery and encourage fair access to AI technologies should be put into place. Closing the access gap to healthcare can be achieved in part by providing funds and resources to develop AI solutions customized to the needs of varied populations.
7.7 Fostering Public Confidence
Increasing public confidence in AI technologies is essential to their broad adoption. Building patient confidence in AI-driven healthcare solutions can be facilitated by open communication about the advantages and constraints of AI as well as patient participation in the decision-making process. Campaigns for education and public participation can help dispel myths about AI and allay worries about its use to medicine.
7.8 Creating Sturdy Assessment Frameworks
To evaluate the impact, safety, and effectiveness of AI systems in healthcare, comprehensive evaluation frameworks are required. To guarantee that AI solutions live up to strict quality and reliability criteria, these frameworks should involve thorough validation and clinical trials. Standardized measurements and standards for AI system evaluation can make comparison easier and boost overall performance.
7.9 Fostering Open-Source Creation
Through encouraging cooperation and creativity, open-source development can hasten the advancement of AI in healthcare. Promoting the creation and exchange of open-source AI datasets and tools can aid in democratizing access to these technologies and advancing their moral application. Researchers and developers may be able to further the use of AI in healthcare by participating in open-source projects.
7.10 Corresponding Regulation and Policy
To create laws and policies that encourage the moral and responsible application of AI in healthcare, legislators and regulatory organizations should collaborate. This entails developing frameworks for algorithmic transparency, data privacy, and accountability to guarantee that AI systems are applied in a way that puts patient safety and wellbeing first. Taking Part in
The creation of unified international standards for AI in healthcare might also be aided by international regulatory organizations.
8. SUGGESTIONS
There are a number of ideas and tactics put out to fully utilize AI in healthcare:
8.1 Improving the Caliber of Data
Purchasing annotated datasets of superior quality is essential.While protecting patient privacy, efforts should be made to standardize data gathering techniques and enhance data sharing throughout healthcare facilities.
8.2 Encouraging Multidisciplinary Cooperation
It is crucial to promote cooperation between AI researchers, medical experts, legislators, and patients. By using an interdisciplinary approach, it will be possible to guarantee that AI technologies are developed with a thorough awareness of both regulatory and clinical requirements.
8.3 Applying Moral Principles
It is essential to create and put into practice ethical rules for AI in healthcare. These rules ought to cover things like accountability, openness, and prejudice. AI systems should undergo routine audits to assist detect and address any ethical issues.
8.4 Encouragement of Ongoing Education
AI training and education should be ongoing for healthcare workers. They will be able to successfully integrate AI tools into their practice and keep current on the most recent developments thanks to this.
8.5 Promoting Creativity
Investments in AI research and development should come from both public and private sectors. Public-private collaborations, grants, and incentives can hasten innovation and expedite the release of innovative AI technologies.
8.6 Guaranteeing Adherence to Regulations
It's imperative to create precise regulatory frameworks for AI in healthcare. In order to guarantee that new technologies fulfill safety and efficacy criteria without impeding innovation, regulatory organizations should collaborate closely with AI developers.
8.7 Encouraging Patient-Centric AI Technologies ought to be created with the requirements and results of patients in mind. Incorporating patients into the development process helps guarantee that AI solutions are intuitive to use and effectively tackle practical issues in healthcare provision.
8.8 Increasing Conscience
Educating the public on the advantages and constraints of artificial intelligence in healthcare helps foster acceptance and confidence. It is crucial to communicate openly about the workings of AI systems and the security measures put in place to secure patient data.
CONCLUSION
With its ability to address some of the most serious issues facing the healthcare industry, artificial intelligence has the potential to completely change the industry. Artificial Intelligence (AI) has the potential to enhance patient outcomes, save costs, and boost the effectiveness of healthcare systems by enabling tailored medication and improving diagnostic accuracy. To fully realize this promise, though, a number of legal, moral, and technological issues must be resolved. We can make sure that AI turns into a useful instrument in the pursuit of better healthcare for everybody by making investments in high-quality data, encouraging interdisciplinary collaboration, putting ethical norms into practice, and encouraging innovation. Although AI in healthcare is still in its infancy, the field is making encouraging strides. Maintaining a patient-centric perspective is crucial as we move forward, making sure that AI technologies are created and applied with the intention of enhancing patient care. AI has the potential to completely transform healthcare, making it more efficient, effective, and fair for all people with the correct approaches and a dedication to moral and responsible use.